Security News

Cybersecurity researchers have disclosed multiple security flaws impacting open-source machine learning (ML) tools and frameworks such as MLflow, H2O, PyTorch, and MLeap that could pave the way...
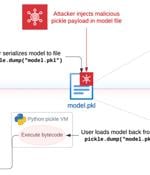
The security risks posed by the Pickle format have once again come to the fore with the discovery of a new "hybrid machine learning (ML) model exploitation technique" dubbed Sleepy Pickle. The...
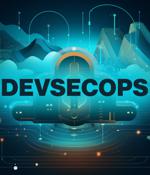
As artificial intelligence advances and machine learning moves to the center of an organization, there's an emerging challenge: how to incorporate ML security into the broad development cycle. That's where machine learning security operations enters the picture.
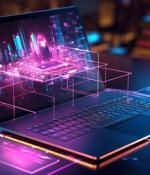
Nearly 70% of businesses said that fraud losses have increased in recent years and most businesses reported that they plan to increase their fraud management budgets by at least 8% to as much as 19%. Despite their plans to increase their fraud prevention budgets, data shows that businesses may not be completely aligned with consumer expectations. Enable real-time fraud detection: Machine learning can help businesses detect and prevent fraud threats in real time, helping to identify both known and unknown threats to stay ahead of fraudsters.
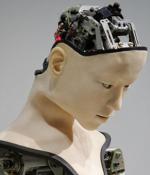
Machine learning models are algorithms that process data to generate meaningful insights and inform critical business decisions. When these data sources contain sensitive or proprietary information, using them for machine learning model training or evaluation/inference raises significant privacy and security concerns.
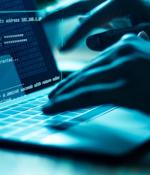
Researchers have trained a ML model to detect keystrokes by sound with 95% accuracy. Abstract: With recent developments in deep learning, the ubiquity of microphones and the rise in online services via personal devices, acoustic side channel attacks present a greater threat to keyboards than ever.
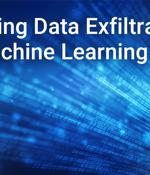
Data exfiltration often serves as the final act of a cyberattack, making it the last window of opportunity to detect the breach before the data is made public or is used for other sinister activities, such as espionage. While prevention of data exfiltration through security controls is ideal, the escalating complexity and dispersion of infrastructures, accompanied by the integration of legacy devices, makes prevention a strenuous task.
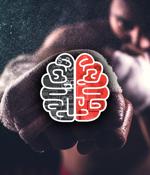
Automated malware campaigns will drastically change the reaction speed of malware gangs. The technology to run malware campaigns and automatically bypass new defenses is most definitely doable nowadays, but thus far, we haven't seen anything of the kind.
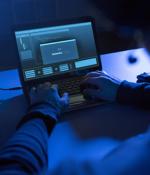
Abstract: Given the computational cost and technical expertise required to train machine learning models, users may delegate the task of learning to a service provider. We show how a malicious learner can plant an undetectable backdoor into a classifier.
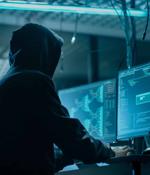
There is a lesson in that similarity: the complex mathematical attacks make for good academic papers, but we mustn't lose sight of the fact that insecure software will be the likely attack vector for most ML systems. At their core, modern ML systems have complex mathematical models that use training data to become competent at a task.