Security News > 2023 > February > Manipulating Weights in Face-Recognition AI Systems
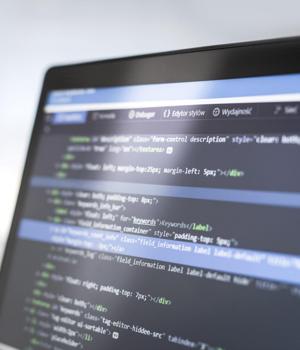
Abstract: In this paper we describe how to plant novel types of backdoors in any facial recognition model based on the popular architecture of deep Siamese neural networks, by mathematically changing a small fraction of its weights.
These backdoors force the system to err only on specific persons which are preselected by the attacker.
We show how such a backdoored system can take any two images of a particular person and decide that they represent different persons, or take any two images of a particular pair of persons and decide that they represent the same person, with almost no effect on the correctness of its decisions for other persons.
We have experimentally verified the attacks on a FaceNet-based facial recognition system, which achieves SOTA accuracy on the standard LFW dataset of 99.35%. When we tried to individually anonymize ten celebrities, the network failed to recognize two of their images as being the same person in 96.97% to 98.29% of the time.
In all of our experiments, the benign accuracy of the network on other persons was degraded by no more than 0.48%. It's a weird attack.
On the one hand, the attacker has access to the internals of the facial recognition system.