Security News > 2022 > October > Inserting a Backdoor into a Machine-Learning System
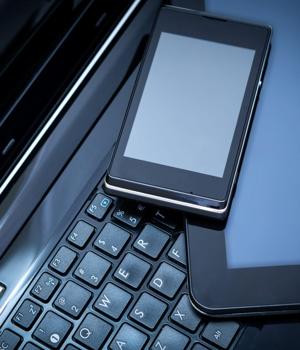
Abstract: Early backdoor attacks against machine learning set off an arms race in attack and defence development.
Defences have since appeared demonstrating some ability to detect backdoors in models or even remove them.
These defences work by inspecting the training data, the model, or the integrity of the training procedure.
In this work, we show that backdoors can be added during compilation, circumventing any safeguards in the data preparation and model training stages.
The trick is for the compiler to recognise what sort of model it's compiling-whether it's processing images or text, for example-and then devising trigger mechanisms for such models that are sufficiently covert and general.
The takeaway message is that for a machine-learning model to be trustworthy, you need to assure the provenance of the whole chain: the model itself, the software tools used to compile it, the training data, the order in which the data are batched and presented-in short, everything.