Security News > 2018 > March > The Malicious Use of Artificial Intelligence in Cybersecurity
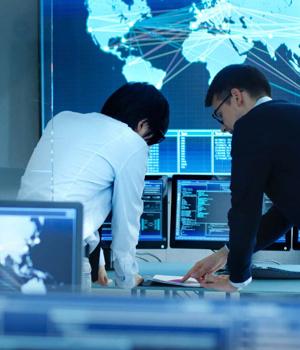
Criminals and Nation-state Actors Will Use Machine Learning Capabilities to Increase the Speed and Accuracy of Attacks Scientists from leading universities, including Stanford and Yale in the U.S. and Oxford and Cambridge in the UK, together with civil society organizations and a representation from the cybersecurity industry, last month published an important paper titled, The Malicious Use of Artificial Intelligence: Forecasting, Prevention, and Mitigation. While the paper (PDF) looks at a range of potential malicious misuses of artificial intelligence (which includes and focuses on machine learning), our purpose here is to largely exclude the military and concentrate on the cybersecurity aspects. It is, however, impossible to completely exclude the potential political misuse given the interaction between political surveillance and regulatory privacy issues. Artificial intelligence (AI) is the use of computers to perform the analytical functions normally only available to humans – but at machine speed. ‘Machine speed’ is described by Corvil’s David Murray as, “millions of instructions and calculations across multiple software programs, in 20 microseconds or even faster.” AI simply makes the unrealistic, real. The problem discussed in the paper is that this function has no ethical bias. It can be used as easily for malicious purposes as it can for beneficial purposes. AI is largely dual-purpose; and the basic threat is that zero-day malware will appear more frequently and be targeted more precisely, while existing defenses are neutralized – all because of AI systems in the hands of malicious actors. Current Machine Learning and Endpoint Protection Today, the most common use of the machine learning (ML) type of AI is found in next-gen endpoint protection systems; that is, the latest anti-malware software. It is called ‘machine learning’ because the AI algorithms within the system ‘learn’ from many millions (and increasing) samples and behavioral patterns of real malware. Detection of a new pattern can be compared with known bad patterns to generate a probability level for potential maliciousness at a speed and accuracy not possible for human analysts within any meaningful timeframe. It works – but with two provisos: it depends upon the quality of the ‘learning’ algorithm, and the integrity of the data set from which it learns. Potential abuse can come in both areas: manipulation or even alteration of the algorithm; and poisoning the data set from which the machine learns. The report warns, “It has been shown time and again that ML algorithms also have vulnerabilities. These include ML-specific vulnerabilities, such as inducing misclassification via adversarial examples or via poisoning the training data… ML algorithms also remain open to traditional vulnerabilities, such as memory overflow. There is currently a great deal of interest among cyber-security researchers in understanding the security of ML systems, though at present there seem to be more questions than answers.” The danger is that while these threats to ML already exist, criminals and nation-state actors will begin to use their own ML capabilities to increase the speed and accuracy of attacks against ML defenses. On data set poisoning, Andy Patel, security advisor at F-Secure, warns, “Diagnosing that a model has been incorrectly trained and is exhibiting bias or performing incorrect classification can be difficult.” The problem is that even the scientists who develop the AI algorithms don’t necessarily understand how they work in the field. He also notes that malicious actors aren’t waiting for their own ML to do this. “Automated content generation can be used to poison data sets. This is already happening, but the techniques to generate the content don't necessarily use machine learning. For instance, in 2017, millions of auto-generated comments regarding net neutrality were submitted to the FCC.” The basic conflict between attackers and defenders will not change with machine learning – each side seeks to stay ahead of the other; and each side briefly succeeds. “We need to recognize that new defenses that utilize technology such as AI may be most effective when initially released before bad actors are building countermeasures and evasion tactics intended to circumvent them,” comments Steve Grobman, CTO at McAfee. Put simply, the cybersecurity industry is aware of the potential malicious use of AI, and is already considering how best to react to it. “Security companies are in a three-way race between themselves and these actors, to innovate and stay ahead, and up until now have been fairly successful,” observes Hal Lonas, CTO at Webroot. “Just as biological infections evolve to more resistant strains when antibiotics are used against them, so we will see malware attacks change as AI defense tactics are used over time.” Hyrum Anderson, one of the authors of the report, and technical director of data science at Endgame, accepts the industry understands ML can be abused or evaded, but not necessarily the methods that could be employed. “Probably fewer data scientists in infosec are thinking how products might be misused,” he told SecurityWeek; “for example, exploiting a hallucinating model to overwhelm a security analyst with false positives, or a similar attack to make AI-based prevention DoS the system.” Indeed, even this report failed to mention one type of attack (although there will undoubtedly be others). “The report doesn’t address the dangerous implications of machine learning based de-anonymization attacks,” explains Joshua Saxe, chief data scientist at Sophos. Data anonymization is a key requirement of many regulations. AI-based de-anonymization is likely to be trivial and rapid. Anderson describes three guidelines that Endgame uses to protect the integrity and secure use of its own ML algorithms. The first is to understand and appropriately limit the AI interaction with the system or endpoint. The second is to understand and limit the data ingestion; for example, anomaly detection that ingests all events everywhere versus anomaly detection that ingests only a subset of ‘security-interesting’ events. In order to protect the integrity of the data set, he suggests, “Trust but verify data providers, such as the malware feeds used for training next generation anti-virus.” The third: “After a model is built, and before and after deployment, proactively probe it for blind spots. There are fancy ways to do this (including my own research), but at a minimum, doing this manually is still a really good idea.” Identity A second area of potential malicious use of AI revolves around ‘identity’. AI’s ability to both recognize and generate manufactured images is advancing rapidly. This can have both positive and negative effects. Facial recognition for the detection of criminal acts and terrorists would generally be consider beneficial – but it can go too far. “Note, for example,” comments Sophos’ Saxe, “the recent episode in which Stanford researchers released a controversial algorithm that could be used to tell if someone is gay or straight, with high accuracy, based on their social media profile photos.” “The accuracy of the algorithm,” states the research paper, “increased to 91% [for men] and 83% [for women], respectively, given five facial images per person.” Human judges achieved much lower accuracy: 61% for men and 54% for women. The result is typical: AI can improve human performance at a scale that cannot be contemplated manually. “Critics pointed out that this research could empower authoritarian regimes to oppress homosexuals,” adds Saxe, “but these critiques were not heard prior to the release of the research.” This example of the potential misuse of AI in certain circumstances touches on one of the primary themes of the paper: the dual-use nature of, and the role of ‘ethics’ in, the development of artificial intelligence. We look at ethics in more detail below. A more positive use of AI-based recognition can be found in recent advances in speech recognition and language comprehension. These advances could be used for better biometric authentication – were it not for the dual-use nature of AI. Along with facial and speech recognition there has been a rapid advance in the generation of synthetic images, text, and audio; which, says the report, “could be used to impersonate others online, or to sway public opinion by distributing AI-generated content through social media channels.” Synthetic image generation in 2014 and 2017 For authentication, Webroot’s Lonas believes we will need to adapt our current authentication approach. “As the lines between machines and humans become less discernible, we will see a shift in what we currently see in authentication systems, for instance logging in to a computer or system. Today, authentication is used to differentiate between various humans and prevent impersonation of one person by another. In the future, we will also need to differentiate between humans and machines, as the latter, with help from AI, are able to mimic humans with ever greater fidelity.” The future potential for AI-generated fake news is a completely different problem, but one that has the potential to make Russian interference in the 2016 presidential election somewhat pedestrian. Just last month, the U.S. indicted thirteen Russians and three companies “for committing federal crimes while seeking to interfere in the United States political system.” A campaign allegedly involving hundreds of people working in shifts and with a budget of millions of dollars spread misinformation and propaganda through social networks. Such campaigns could increase in scope with fewer people and far less cost with the use of AI. In short, AI could be used to make fake news more common and more realistic; or make targeted spear-phishing more compelling at the scale of current mass phishing through the misuse or abuse of identity. This will affect both business cybersecurity (business email compromise, BEC, could become even more effective than it already is), and national security. The Ethical Problem The increasing use of AI in cyber will inevitably draw governments into the equation. They will be concerned about more efficient cyber attacks against the critical infrastructure, but will also become embroiled over civil society concerns over their own use of AI in mass surveillance. Since machine learning algorithms become more efficient with the size of the data set from which they learn, the ‘own it all’ mentality exposed by Edward Snowden will become increasingly compelling to law enforcement and intelligence agencies. The result is that governments will be drawn into the ethical debate about AI and the algorithms it uses. In fact, this process has already started, with the UK’s financial regulator warning that it will be monitoring the use of AI in financial trading. Governments seek to assure people that its own use of citizens’ big data will be ethical (relying on judicial oversight, court orders, minimal intrusion, and so on). It will also seek to reassure people that business makes ethical use of artificial intelligence – GDPR has already made a start by placing controls over automated user profiling. While governments often like the idea of ‘self-regulation’ (it absolves them from appearing to be over-proscriptive), ethics in research is never adequately covered by scientists. The report states the problem: “Appropriate responses to these issues may be hampered by two self-reinforcing factors: first, a lack of deep technical understanding on the part of policymakers, potentially leading to poorly-designed or ill-informed regulatory, legislative, or other policy responses; second, reluctance on the part of technical researchers to engage with these topics, out of concern that association with malicious use would tarnish the reputation of the field and perhaps lead to reduced funding or premature regulation.” There is a widespread belief among technologists that politicians simply don’t understand technology. Chris Roberts, chief security architect at Acalvio, is an example. “God help us if policy makers get involved,” he told SecurityWeek. “Having just read the last thing they dabbled in, I’m dreading what they’d come up with, and would assume it’ll be too late, too wordy, too much crap and red tape. They’re basically five years behind the curve.” The private sector is little better. Businesses are duty bound, in a capitalist society, to maximize profits for their shareholders. New ideas are frequently rushed to market with little thought for security; and new algorithms will probably be treated likewise. Oliver Tavakoli, CTO at Vectra, believes that the security industry is obligated to help. “We must adopt defensive methodologies which are far more flexible and resilient rather than fixed and (supposedly) impermeable,” he told SecurityWeek. “This is particularly difficult for legacy security vendors who are more apt to layer on a bit of AI to their existing workflow rather than rethinking everything they do in light of the possibilities that AI brings to the table.” “The security industry has the opportunity to show leadership with AI and focus on what will really make a difference for customers and organizations currently being pummeled by cyberattacks,” agrees Vikram Kapoor, co-founder and CTO at Lacework. His view is that there are many areas where the advantages of AI will outweigh the potential threats. “For example,” he continued, “auditing the configuration of your system daily for security best practices should be automated – AI can help. Continuously checking for any anomalies in your cloud should be automated – AI can help there too.” It would probably be wrong, however, to demand that researchers limit their research: it is the research that is important rather than ethical consideration of potential subsequent use or misuse of the research. The example of Stanford’s sexual orientation algorithm is a case in point. Google mathematician Thomas Dullien (aka Halvar Flake on Twitter) puts a common researcher view. Commenting on the report, he tweeted, “Dual-use-ness of research cannot be established a-priori; as a researcher, one usually has only the choice to work on ‘useful’ and ‘useless’ things.” In other words, you cannot – or at least should not – restrict research through imposed policy because at this stage, its value (or lack of it) is unknown. McAfee’s Grobman believes that concentrating on the ethics of AI research is the wrong focus for defending against AI. “We need to place greater emphasis on understanding the ability for bad actors to use AI,” he told SecurityWeek; “as opposed to attempting to limit progress in the field in order to prevent it.” Summary The Malicious Use of Artificial Intelligence: Forecasting, Prevention, and Mitigation makes four high-level recommendations “to better forecast, prevent, and mitigate” the evolving threats from unconstrained artificial intelligence. They are: greater collaboration between policymakers and researchers (that is, government and industry); the adoption of ethical best practices by AI researchers; a methodology for handling dual-use concerns; and an expansion of the stakeholders and domain experts involved in discussing the issues. Although the detail of the report makes many more finely-grained comments, these high-level recommendations indicate there is no immediately obvious solution to the threat posed by AI in the hands of cybercriminals and nation-state actors. Indeed, it could be argued that there is no solution. Just as there is no solution to the criminal use of encryption – merely mitigation – perhaps there is no solution to the criminal use of AI – just mitigation. If this is true, defense against the criminal use of AI will be down to the very security vendors that have proliferated the use of AI in their own products. It is possible, however, that the whole threat of unbridled artificial intelligence in the cyber world is being over-hyped. F-Secure’s Patel comments, “Social engineering and disinformation campaigns will become easier with the ability to generate ‘fake’ content (text, voice, and video). There are plenty of people on the Internet who can very quickly figure out whether an image has been photoshopped, and I’d expect that, for now, it might be fairly easy to determine whether something was automatically generated or altered by a machine learning algorithm. “In the future,” he added, “if it becomes impossible to determine if a piece of content was generated by ML, researchers will need to look at metadata surrounding the content to determine its validity (for instance, timestamps, IP addresses, etc.).” In short, Patel’s suggestion is that AI will simply scale, in quality and quantity, the same threats that are faced today. But AI can also scale and improve the current defenses against those threats. “The fear is that super powerful machine-learning-based fuzzers will allow adversaries to easily and quickly find countless zero-day vulnerabilities. Remember, though, that these fuzzers will also be in the hands of the white hats… In the end, things will probably look the same as they do now.” Related: Researchers Poison Machine Learning Engines Related: The Role of Artificial Intelligence in Cyber Security Related: Threat Hunting with Machine Learning, AI, and Cognitive Computing (function() { var po = document.createElement("script"); po.type = "text/javascript"; po.async = true; po.src = "https://apis.google.com/js/plusone.js"; var s = document.getElementsByTagName("script")[0]; s.parentNode.insertBefore(po, s); })(); Tweet Kevin Townsend is a Senior Contributor at SecurityWeek. He has been writing about high tech issues since before the birth of Microsoft. For the last 15 years he has specialized in information security; and has had many thousands of articles published in dozens of different magazines – from The Times and the Financial Times to current and long-gone computer magazines.Previous Columns by Kevin Townsend:Statistics Say Don't Pay the Ransom; but Cleanup and Recovery Remains CostlyThe Top Vulnerabilities Exploited by CybercriminalsMcAfee Enhances Product Portfolio, Unveils New Security Operations CentersFormer Barclays CISO to Head WEF's Global Center for Cybersecurity Ransomware Hits City of Atlanta sponsored links Tags: NEWS & INDUSTRY Malware Vulnerabilities