Security News > 2023 > February > Putting Undetectable Backdoors in Machine Learning Models
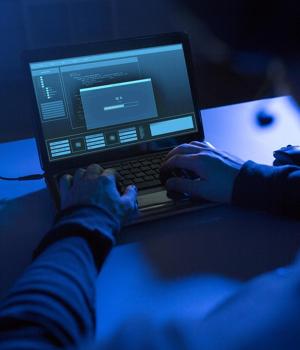
Abstract: Given the computational cost and technical expertise required to train machine learning models, users may delegate the task of learning to a service provider.
We show how a malicious learner can plant an undetectable backdoor into a classifier.
We demonstrate two frameworks for planting undetectable backdoors, with incomparable guarantees.
Second, we demonstrate how to insert undetectable backdoors in models trained using the Random Fourier Features learning paradigm.
We show a similar white-box undetectable backdoor for random ReLU networks based on the hardness of Sparse PCA. Our construction of undetectable backdoors also sheds light on the related issue of robustness to adversarial examples.
In particular, by constructing undetectable backdoor for an "Adversarially-robust" learning algorithm, we can produce a classifier that is indistinguishable from a robust classifier, but where every input has an adversarial example! In this way, the existence of undetectable backdoors represent a significant theoretical roadblock to certifying adversarial robustness.