Security News > 2021 > May > Microsoft: This clever open-source technique helps to protect your privacy
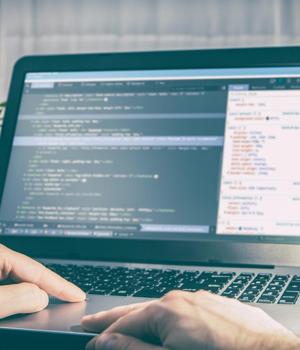
"You only want to learn the larger patterns in the data, and so what differential privacy is doing is adding some noise to hide those smaller patterns that you didn't want to know anyway," Bird explained.
Others reach out to the SmartNoise team on GitHub, which has led to a more formal early adoption programme where Microsoft is helping organisations like Humana and the Educational Results Partnership build differential privacy into research programmes looking at health and education data.
Microsoft has also used differential privacy to share US broadband usage data with researchers looking at how connectivity has affected access to education during the pandemic.
"In Windows telemetry, it's the same type of data and analysis coming over and over and over and over again. Work done once is heavily reused. For operational analytics like telemetry, you're allowing more people to leverage data with privacy guarantees. In machine learning, where it's worth the effort to spend longer training the model or more carefully featurise, to have that privacy guarantee."
Generating synthetic data with differential privacy is most useful if you know the questions you want to ask the data, so you can generate data that successfully answers those questions and preserves those properties in the original data set.
Microsoft customers who don't have the data science expertise to work with the SmartNoise toolkit will eventually see differential privacy as a data-processing option in platforms like Power BI and Azure Data Share, Bird suggested.