Security News > 2021 > May > DefakeHop: A deepfake detection method that tackles adversarial threat detection and recognition
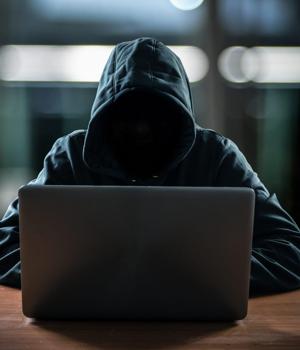
Army researchers developed a deepfake detection method that will allow for the creation of state-of-the-art soldier technology to support mission-essential tasks such as adversarial threat detection and recognition.
Researchers at the U.S. Army Combat Capabilities Development Command, known as DEVCOM, Army Research Laboratory, in collaboration with Professor C.-C. Jay Kuo's research group at the University of Southern California, set out to tackle the significant threat that deepfake poses to our society and national security.
Deepfake refers to artificial intelligence-synthesized, hyper-realistic video content that falsely depicts individuals saying or doing something, said ARL researchers Dr. Suya You and Dr. Shuowen Hu. Most state-of-the-art deepfake video detection and media forensics methods are based upon deep learning, which have many inherent weaknesses in terms of robustness, scalability and portability.
Combining team member experience with machine learning, signal analysis and computer vision, the researchers developed an innovative theory and mathematical framework, the Successive Subspace Learning, or SSL, as an innovative neural network architecture.
Most current state-of-the-art techniques for deepfake video detection and media forensics methods are based on the deep learning mechanism, You said.
This research provides a robust spatial-spectral representation to purify the adversarial inputs, thus adversarial perturbations can be effectively and efficiently defended against.
News URL
http://feedproxy.google.com/~r/HelpNetSecurity/~3/9D3NvDWWgmc/